📢SUPERVISING FRONTIER AI DEVELOPERS: A CRITICAL ANALYSIS
- PCV LLC
- Feb 20
- 2 min read
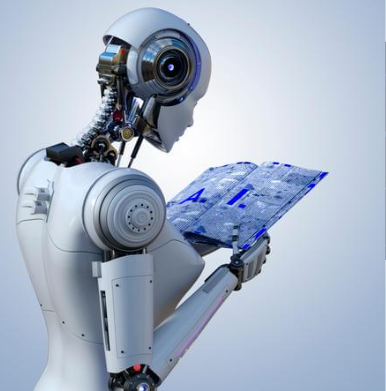
Artificial Intelligence (AI) systems present both transformative benefits and significant risks. The regulatory challenge lies in harnessing AI’s potential while mitigating its dangers. In Supervising Frontier AI Developers, Peter Wills argues that supervision—a regulatory approach with roots in financial oversight—is best suited for managing frontier AI developers like OpenAI, Anthropic, Google DeepMind, and Meta. This blog post explores the key arguments of the paper, the concept of supervision, and its implications for AI governance.
What Does the Paper Address?
The paper examines how regulatory frameworks can effectively oversee AI developers working at the “frontier”—where innovation is most advanced but risks are highest.
The paper critiques existing regulatory tools, arguing that traditional models such as ex post enforcement, predefined rules, or mandatory insurance fail to adequately address the dynamic risks posed by AI. Instead, supervision offers a nuanced, real-time regulatory mechanism that balances innovation with oversight.
What is Supervision?
Supervision is a regulatory approach that grants authorities close, continuous insight into regulated entities’ operations. Unlike rule-based regulations that prescribe strict boundaries, supervision allows for:
Information-gathering: Regulators obtain non-public data from AI developers
Discretionary enforcement: Supervisors can impose consequences proportionate to risks
Proactive intervention: Regulators can prevent risks from materialising rather than responding after harm occurs
Adaptive oversight: Supervision evolves alongside AI developments without stifling innovation
Originating in financial regulation, supervision has been a key mechanism for managing industries that require flexible, real-time governance due to their complexity and sensitivity.
The Four-Part Argument for Supervision
The paper structures the case for supervision through four key arguments:
Defining Supervision as a Regulatory Modality
Supervision differs from rule-based or market-driven approaches by emphasising continuous regulatory engagement
It has been successful in financial regulation, offering lessons for AI governance
Regulatory Objectives for Frontier AI
Frontier AI systems pose unique risks: misuse, concentration of power, and economic disruption
Regulation must address these risks while preserving AI’s benefits
Limitations of Alternative Regulatory Tools
Traditional regulatory models struggle to keep pace with AI’s rapid evolution
Purely rule-based or punitive approaches risk either overregulation or regulatory gaps
Supervision’s Role in Achieving Regulatory Goals
Supervision enhances state capacity by improving intelligence on AI risks
It enables both indirect and direct interventions to manage AI developers’ actions
While supervision is not foolproof, it provides a more effective oversight mechanism than static rules or post-hoc enforcement

Key Considerations and Potential Challenges
The paper acknowledges that supervision is not without risks:
Regulatory Capture: AI firms may influence regulators, reducing effectiveness
Mission Creep: Supervisors may overreach, stifling legitimate innovation
Information Security Risks: Increased government access to AI systems raises concerns about data protection
Addressing these challenges requires transparency, institutional safeguards, and a well-resourced supervisory framework.
Conclusion
The argument of the paper for supervision as the optimal regulatory approach for frontier AI is compelling. By leveraging real-time oversight, discretion, and adaptive governance, supervision can help mitigate AI’s risks without stifling progress. However, careful implementation is crucial to prevent regulatory failures. Policymakers must design supervision mechanisms that are resistant to capture, appropriately scoped, and capable of evolving with AI technology.
As frontier AI development accelerates, the debate over supervision will become increasingly relevant. The question remains: How can governments implement supervision effectively while maintaining public trust and fostering innovation? The answer will shape the future of AI regulation.
Comments